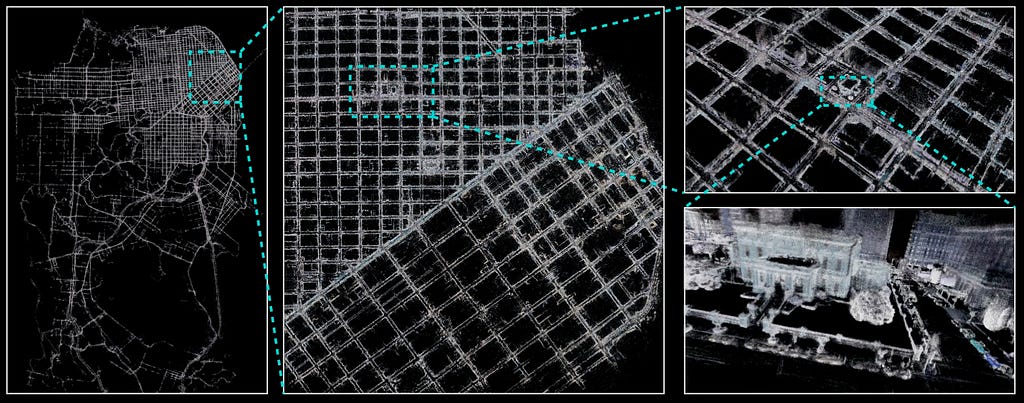
By Luca Del Pero, Engineering Manager; Hugo Grimmett, Level 5 Product Manager; and Peter Ondruska, Head of AV Research
Dodging a pothole. Slamming on the brakes for an unexpected pedestrian. Deciding when it’s safe to enter a busy intersection. Drivers all over the world face unplanned scenarios daily and often must make split-second decisions to ensure safety.
But as autonomous vehicles (AVs) become a mainstream transportation option, the need to make such real-time assessments is no longer isolated to human drivers. To accelerate the development of self-driving technology, Lyft is tapping into our greatest asset — our nationwide rideshare network that is accessible to 95% of the United States population, making it one of the largest in the world.
Every day, trips are completed on our network that cover a wide variety of driving scenarios, ranging from pickups and drop-offs to situations that require immediate and critical thinking — like a car running a red light. To expand our knowledge of how human drivers behave in real-world situations, a subset of cars on our network are equipped with low-cost camera sensors that capture the scenarios drivers face daily.
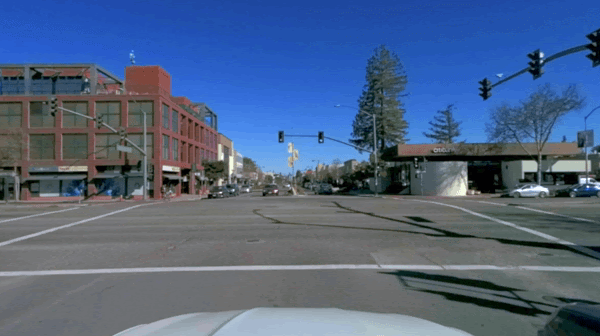
This data enables us to tackle some of the hardest problems in self-driving, from building accurate and up-to-date 3D maps, to understanding human driving patterns, to increasing the sophistication of our simulation tests by having access to rare real-world driving situations. By leveraging this data, Lyft is uniquely positioned to develop safe, efficient, and intuitive self-driving systems.
Using our network to build 3D maps
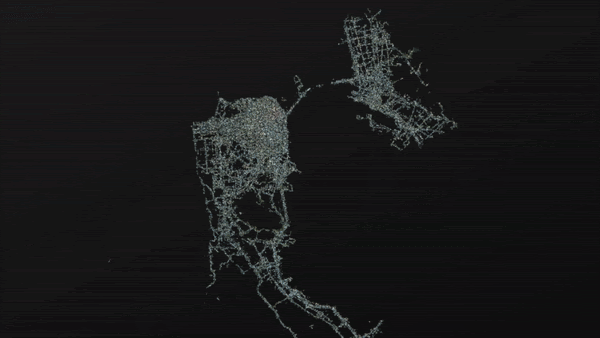
Self-driving cars need accurate 3D models of the environment to properly navigate the complex world around them. Using rideshare data, we’ve built city-scale 3D geometric maps using technology developed by Blue Vision Labs, which we acquired in 2018. While mapping operations teams can build 3D geometric maps for AVs, keeping them up-to-date and scaling their scope is a challenge. We’ve mapped thousands of miles thanks to the wide geographic coverage of the cars on our network. We’re able to continuously update our maps based on a constant stream of data that is immediately logged when a ride is completed.
3D maps for AVs must also contain context about the environment in which the self-driving car will operate, such as information on lanes and traffic lights. Lyft generates this information from our rideshare data by using a combination of 3D computer vision and machine learning to automatically identify traffic objects. The situational data we get from this — like where lanes are and which traffic light drivers must follow — gives us the context we need to understand how drivers handle risky situations, like a driver running a particular red light.
Learning to drive from human trajectories
In addition to empowering us to build 3D geometric maps, our rideshare network helps us better understand human driving patterns. Thanks to our visual localization technology, we’re able to track the real-world trajectories that Lyft drivers follow on our geometric maps with great accuracy. One of the ways we’re using this data is to help our AVs maintain the optimal location in their lane.
While common sense suggests that staying close to the center of the lane is the safest option, historical rideshare data proves that this assumption is not always true. Human driving is much more nuanced due to local features in the road (like parked cars or potholes) and other facets, such as road design or road shape and visibility.
Thanks to ridesharing data, our AV’s motion planner does not need to use ad-hoc heuristics like following lane centers when deciding where to drive, which requires various exceptions to handle all possible corner cases. Instead, the planner can rely on the real-world information and the human driving experience that are naturally encoded in the rideshare trajectories.
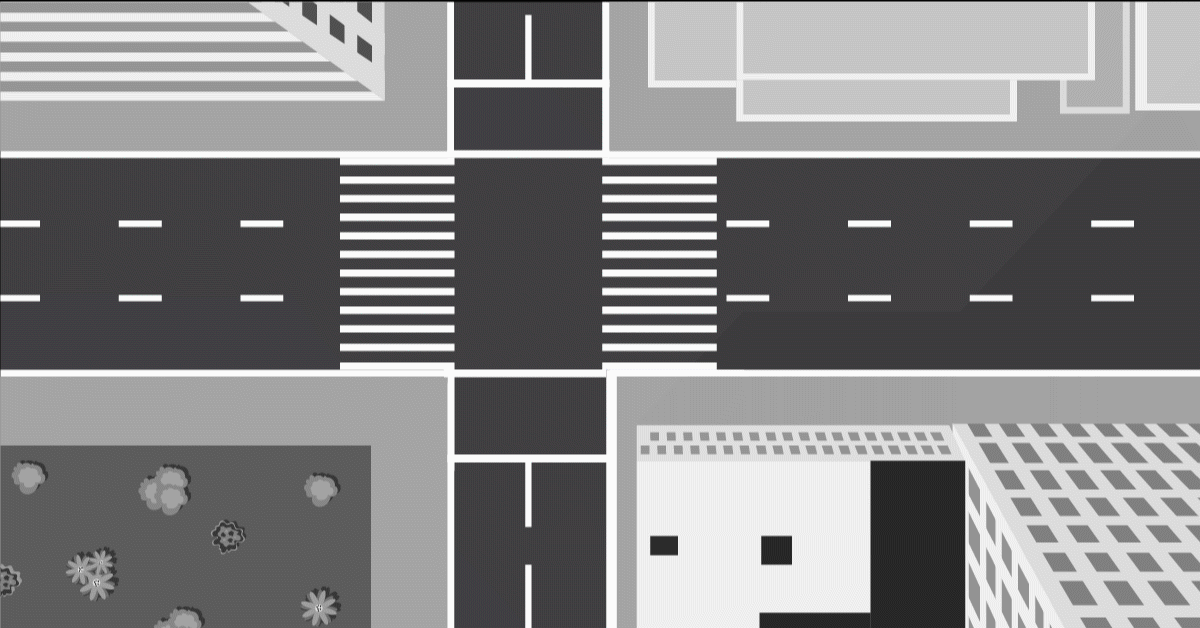
Learning from real-world scenarios for intelligent, autonomous reactions
There are many challenges to AV motion planning beyond determining where to drive within a lane. Complete AV motion planning requires predicting the motion of other traffic agents and responding to unpredictable behaviors, like a driver running a red light.
Data from trips on the Lyft network provides us with the insights necessary to predict how vehicles and other traffic agents will respond in a variety of scenarios. For example, we leverage cases of human drivers being cut off to learn an appropriate deceleration profile. This enables our AVs to respond safely in similar situations. In addition, leveraging historical data from the network results in more comfortable and natural rides compared to a traditional expert system approach.
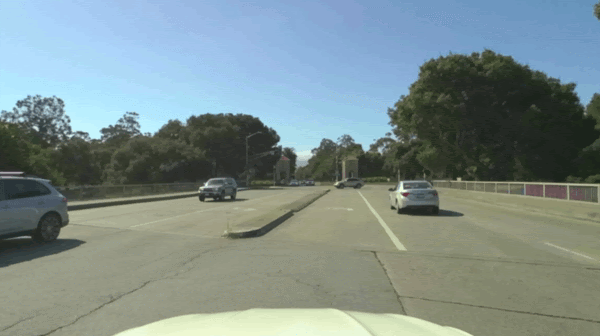
Navigating the road ahead
Building 3D geometric maps, understanding human driving patterns, and learning from human reactions are just three of many examples of how we can use Lyft rideshare data to accelerate AV development. Thanks to this wealth of historical, real-world driving behaviors, Lyft can now tackle the hardest problems in motion planning and prediction with a data-driven approach. With its volume and its diversity, the data from the Lyft network provides the fuel needed by modern machine learning methods, which are hungry for realistic examples of the rare and complex scenarios the AVs will need to face.
This is what’s most unique about Lyft. We aren’t solely building our technology based on previous AV missions or human-created simulations. We’re leveraging data derived from one of the largest rideshare networks in the world to accelerate autonomous driving technology while ensuring a safe and secure AV experience for all Lyft riders, both now and in the future.
If you’re interested in learning more about how Lyft is navigating the autonomous road ahead, follow @LyftLevel5 on Twitter and this blog for more technical content.
Accelerating Autonomous Driving with Lyft’s Ridesharing Data was originally published in Lyft Level 5 on Medium, where people are continuing the conversation by highlighting and responding to this story.