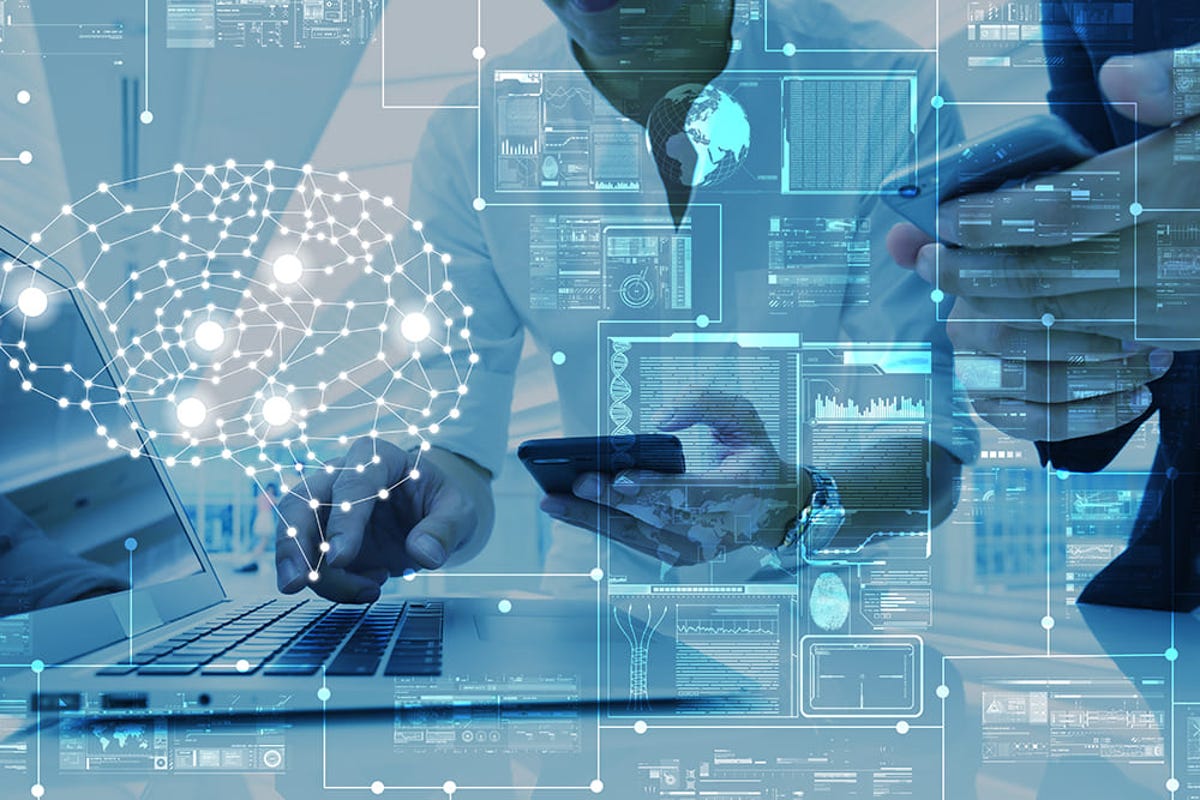
Shutterstock
NYU Professor Emeritus Gary Marcus, a frequent critic of the hype that surrounds artificial intelligence, recently sat down with ZDNET to offer a rebuttal to remarks by Yann LeCun, Meta’s chief AI scientist, in a ZDNET interview with LeCun in September.
LeCun had cast doubt on Marcus’ argument in favor of symbol manipulation as a path to more sophisticated AI. LeCun also remarked that Marcus had no peer-reviewed papers in AI journals.
Marcus has, in fact, published peer-reviewed papers, a list of which appears in context in the interview below. But Marcus’ rebuttal deals more substantively with the rift between the two, who have sparred with one another on social media for years.
“There’s a space of possible architectures for AI,” says NYU Professor Emeritus Gary Marcus. “Most of what we’ve studied is in one little tiny corner of that space.”
Gary Marcus
Marcus claims LeCun has not really engaged with Marcus’ ideas, simply dismissing them. He argues, too, that LeCun has not given other scholars a fair hearing, such as Judea Pearl, whose views about AI and causality form a noteworthy body of work.
Marcus argues LeCun’s behavior is part of a pattern of deep learning researchers dismissing peers from outside of deep learning who voice criticism or press for other avenues of inquiry.
“You have some people who have a ton of money, and a bunch of recognition, who are trying to crowd other people out,” Marcus said of LeCun and other deep learning scholars. They are, he said, borrowing a term from computational linguist Emily Bender, “sucking the oxygen from the room” by not engaging with competing ideas.
The rift between Marcus and LeCun, in Marcus’s view, is odd given that Marcus contends LeCun has finally come around to agreeing with many criticisms Marcus has made for years.
“It basically seemed like he was saying that all the things that I had said, which he had said were wrong, were the truth,” said Marcus. Marcus has expressed his strong views on deep learning both in books, the most recent being 2019’s Rebooting AI, with Ernie Davis, although there are elements in a much earlier work, The Algebraic Mind; and in numerous papers, including his most extensive critique, in 2018, “Deep Learning: A Critical Appraisal.”
In fact, the points of common ground between the two scholars are such that, “In a different world, LeCun and I would be allies,” Marcus said.
Also: Meta’s AI guru LeCun: Most of today’s AI approaches will never lead to true intelligence
“The No. 1 point on which LeCun and I are in alignment is that scaling alone is not enough,” said Marcus, by which he means that making ever-larger versions of neural nets such as GPT-3 will not, in and of itself, lead to the kind of intelligence that matters.
There also remain fundamental disagreements between the two scholars. Marcus has, as far back as The Algebraic Mind, argued passionately for what he calls “innateness,” something that is wired into the mind to give structuring to intelligence.
“My view is if you look at biology that we are just a huge mix of innate structure,” Marcus said. LeCun, he said, would like everything to be learned.
“I think the great irony is that LeCun’s own greatest contribution to AI is the innate prior of convolution, which some people call translation invariance,” said Marcus, alluding to convolutional neural networks.
The one thing that is bigger than either researcher, and bigger than the dispute between them, is that AI is at an impasse, with no clear direction to achieving the kind of intelligence the field has always dreamed of.
“There’s a space of possible architectures for AI,” said Marcus. “Most of what we’ve studied is in one little tiny corner of that space; that corner of the space is not quite working. The question is, How do we get out of that corner and start looking at other places?”
What follows is a transcript of the interview edited for length.
If you’d like to dip into Marcus’s current writing on AI, check out his Substack.
ZDNET: This conversation is in response to the recent ZDNET interview with Yann LeCun of Meta Properties in which you were mentioned. And so, first of all, what is important to mention about that interview with LeCun?
Gary Marcus: LeCun’s been critiquing me a lot lately, in the ZDNET interview, in an article in Noema, and on Twitter and Facebook, but I still don’t know how much LeCun has actually read of what I’ve said. And I think part of the tension here is that he has sometimes criticized my work without reading it, just on the basis of things like titles. I wrote this 2018 piece, “Deep Learning: A Critical Appraisal,” and he smacked it down, publicly, the first chance he got on Twitter. He said it was “mostly wrong.” And I tried to push him on what about it was wrong. He never said.
Artificial Intelligence
I believe that he thinks that that article says that we should throw away deep learning. And I’ve corrected him on that numerous times. He again made that error [in the ZDNET interview]. If you actually read the paper, what it says is that I think deep learning is just one tool among many, and that we need other things as well.
So anyway, he attacked this paper previously, and he’s a big senior guy. At that time [2018], he was running Facebook AI. Now he’s the chief AI scientist at Facebook and a vice president there. He is a Turing Award winner. So, his words carry weight. And when he attacks somebody, people follow suit.
Of course, we don’t all have to read each other’s articles, but we shouldn’t be saying they’re mostly wrong unless we’ve read them. That’s not really fair. And to me it felt like a little bit of an abuse of power. And then I was really astounded by the interview that you ran with him because it sounded like he was arguing for all the things I had put out there in that paper that he ridiculed: We’re not going to get all the way there, at least with current deep learning techniques. There were many other, kind of, fine points of overlap such that it basically seemed like he was saying that all the things that I had said, which he had said were wrong, were the truth.
And that would be, sort of, irritating enough for me — no academic likes to not be cited — but then he took a pot shot at me and said that I’d never published anything in a peer-reviewed AI journal. Which isn’t true. He must not have fact-checked that. I’m afraid you didn’t either. You kindly corrected it.
ZDNET: I apologize for not fact-checking it.
[Marcus points out several peer-reviewed articles in AI journals: Commonsense Reasoning about Containers using Radically Incomplete Information in Artificial Intelligence; Reasoning from Radically Incomplete Information: The Case of Containers in Advances In Cog Sys; The Scope and Limits of Simulation in Automated Reasoning in Artificial Intelligence; Commonsense Reasoning and Commonsense Knowledge in Communications of the ACM; Rethinking eliminative connectionism, Cog Psy)]
GM: This stuff happens. I mean, part of it, it’s like an authority says something and you just believe it. Right. I mean, he’s Yann LeCun.
ZDNET: It should be fact-checked. I agree with you.
GM: Anyway. He said it. I corrected him. He never apologized publicly. So, anyway, what I saw there, the combination of basically saying the same things that I’ve been saying for some time, and attacking me, was part of a repositioning effort. And I really lay out the case for that in this Substack piece: “How New Are Yann LeCun’s ‘New’ Ideas?“
And the case I made there is that he’s, in fact, trying to rewrite history. I gave numerous examples; as they say nowadays, I brought receipts. People who are curious can go read it. I don’t want to repeat all the arguments here, but I see this on multiple dimensions. Now, some people saw that and were like, “Will LeCun be punished for this?” And, of course, the answer is, no, he won’t be. He’s powerful. Powerful people are never punished for things, or rarely.
Also: Resisting the urge to be impressed; what we talk about when we talk about AI
But there’s a deeper set of points. You know, aside from me personally being pissed and startled, I’m not alone. I gave one example [in the Substack article] of [Jürgen] Schmidhuber [adjunct professor at IDSIA Dalle Molle Institute for Artificial Intelligence] feeling the same way. It came out in the intervening week that Judea Pearl, who is also a Turing Award winner like Yann, also feels that his work has not been mentioned by the mainstream machine learning community, either. Pearl said this in a pretty biting way, saying, “LeCun’s been nasty to Marcus but he hasn’t even bothered to mention me,” is more or less what Pearl said. And that’s pretty damning that one Turing Award winner doesn’t even cite the other
LeCun is thinking about causality, and we all know that the leader in causality is Pearl. That doesn’t mean Pearl has solved all of the problems, but he has done more to call attention to why it’s important to machine learning than anyone else. He’s contributed more, kind of, formal machinery to it. I don’t think he’s solved that problem, but he has broken open that problem. [For LeCun] to say, I’m going to build world models, well, world models are about understanding causality, and to neglect Pearl is shocking.
And it is part of a style of “Not invented here.” Now, an irony is, I think probably everything that LeCun said in your interview — not the stuff about me, but about this kind of state of the field — he probably came to on his own. I don’t think he plagiarized it from me. And I say that in the [Substack] article. But, why wait four years to find this stuff out when your NYU neighbor can have something to say.
Marcus has been an unrelenting critic of deep learning, most forcefully in his 2019 book with NYU colleague Ernest Davis, Rebooting AI. The duo argue that the lack of common sense in machine learning programs is one of the biggest factors in the potential harm those programs may cause.
Gary Marcus
He also had a huge fight with Timnit Gebru [former Google researcher and now founder and executive director of the Distributed Artificial Intelligence Research Institute, DAIR] a couple of years ago on Twitter — you can look that up if you’d like — such that he [LeCun] actually left Twitter. He bullied Timnit. He, I think, downplays Schmidhuber’s contributions. He downplays Pearl’s. So, like a lot of people who want to defend the honor of the ways in which machine learning is done right now, he kind of demonized me. And you saw that in [the ZDNET interview] he attacked me pretty directly.
In my view, it’s all part of the larger thing, which is that you have some people who have a ton of money, and a bunch of recognition, who are trying to crowd other people out. And they’re not really recognizing the irony of this because they themselves were crowded out of until around 2012. So they had really good ideas, and their really good ideas didn’t look so good in 2010. My favorite quote about this still belongs to Emily Bender. She said, the problem with this is that they’re sucking the oxygen from the room, they’re making it hard for other people to pursue other approaches, and they’re not engaging those approaches.
There’s a whole field of neuro-symbolic AI that LeCun is not engaging with, sometimes bashes as being incoherent; when I advocated for it in 2018, he said that it was “mostly wrong.” But he never actually engages with the work. And this is not seemly for someone of his stature to do that. You know, it’s fine for him to disagree with it and say, “I would do it in this other, better way or these premises are false.” But he doesn’t engage it.
There was a wonderful tweet […] on a different topic by Mikell Taylor, who’s a roboticist, and she said a bunch of these fans of Tesla are basically saying, Well, why don’t you deal with it? And her point was, Well, nobody can do the things that Tesla is promising right now. And nobody can do the things that deep learning is meant to do right now. The reality is, these things have been oversold.
We don’t have in 2022 the technological readiness to power a domestic robot to be able to understand the world. We’re still failing at driverless cars. We have these chat bots that are sometimes great and sometimes absolutely foolish. And my view is, it’s like we’re on K2, we’ve climbed this incredible mountain, but it turns out it’s the wrong mountain. Some of us have been pointing that out for a while, LeCun is recognizing now that it’s not the correct mountain.
Also: OpenAI has an inane text bot, and I still have a writing job
Taylor’s point is, it’s legitimate to criticize something even if you don’t have a better solution. Sometimes the better solutions just really aren’t at hand. But you still need to understand what’s gone wrong right now. And LeCun wants it both ways because he doesn’t actually have the solution to these problems either. He’s now going around giving a talk, saying, I see that the field is a mess. Around the same day as that interview was posted, he gave a talk in which he said, ML sucks. Of course, if I said that people would, like, slash my tires, but he can say it because he’s LeCun.
He says ML sucks, and then he has some vague noises about how he’ll solve it. An interesting manifesto paper (“A Path Towards Autonomous Machine Intelligence“) that he wrote this summer that involves multiple modules, including a kind of configurable predictor. The point is, [LeCun’s new approach] is not really an implemented theory either. It’s not like LeCun can go home and say, “All these things that Marcus worried about, and that I’m now worried about, are solved with this.” All he can say is, “I have an instinct that we might go this way.”
I think there is something to saying we need richer models of the world. In fact, that’s what I’ve been saying for years. So, for example, one of the peer-reviewed articles that I happen to have in AI journals is a model of how you understand what happens in a container, which is a very interesting thing because a lot of what we do in the world is actually deal with containers.
So, on my desk right now, I have one container that holds pens and pencils and stuff like that, and I have another that has a glass of water in it. I know things about them, like, if I take something out, it’s not in the container anymore. If I tip over the container, everything will fall out. We can do all kinds of physical reasoning about containers. We know that if we had a coffee cup with holes in it, and I pour in the coffee, then the coffee would spill out.
Ernie Davis, who’s an NYU colleague of LeCun’s, and I wrote that paper in Artificial Intelligence, one of the leading journals in the field, where we give a classic formal logic account of this. And LeCun, in his interview with you, was talking about physical reasoning in common sense circumstances. So here is a perfect example of a possible [alternative] theory which Davis and I proposed. I don’t think that the theory that Davis and I proposed is right, to be honest. I think it kind of frames up the problem. But it’s a hard problem and there’s room to do more work on it. But the point is, it’s not like LeCun has actually got an implemented theory of physical reasoning over containers that he can say is an alternative. So he points to me and says, Well, you don’t have an alternative. Well, he doesn’t have an alternative to the thing that I proposed.
Also: What’s next for AI: Gary Marcus talks about the journey toward robust artificial intelligence
You don’t get good science when what people are doing is attacking people’s credentials. Francis Crick wasn’t a biologist. Does that mean that his model of DNA is wrong? No. He was a physicist, but you can come from another field and have something to say. There are many, many examples of that historically.
If you suck the oxygen [out of] the room by bullying other people out of alternative hypotheses, you run the risk of having the wrong idea. There’s a great historical precedent of this, great and sad, a clear one, which is in the early 1900s, most of the people in the field thought that genes, which Mendel had discovered, were made of proteins. They were looking for the molecular basis of genes, and they were all wrong. And they wrote proud articles about it. Somebody won a Nobel Prize for, I think it was in 1946, for the tobacco virus, which they thought was a protein and wasn’t actually. It’s one of the few Nobel prizes that was actually wrongly awarded. And turns out that DNA is actually an acid, this weird thing called DNA that people didn’t know much about at the time. So, you get these periods in history where people are very clear about what the answer is and wrong.
In the long term, science is self-correcting. But the reason that we have a kind of etiquette and best practice about no ad hominem, cite other people’s work, build upon it, is so that we don’t have mistakes like that and so that we can be more efficient. If we’re dismissive, and that’s really the word I would most use around LeCun, if we are dismissive of other people’s work, like Judea Pearl’s work, my work, Schmidhuber’s work, the whole neuro-symbolic community, we risk dwelling on the wrong set of models for too long.
ZDNET: Regarding your 2018 paper, which is a wonderful article, the key quote for me is, “Deep learning thus far is shallow, it has limited capacity for transfer, although deep learning is capable of some amazing things.” We’re all kind of enamored of the amazing things, meaning it morphs our photographs in our high-resolution smartphone pictures. Let’s be frank: This stuff works on some level. And now you and LeCun are both saying this is not intelligence, and it’s not even a beginning of intelligence, it’s really primitive. You’re both up against, it seems to me, an industrial regime that is more and more profiting from putting forward these amazing things that these machines do.
GM: The first thing I’ll say is, I don’t want to quibble over whether it is or is not intelligence. That depends on how you define the terms. So, I would say it’s not unreasonable to call deep learning a form of intelligence, depending on your definition. You might call a calculator intelligent if you want to, or a chess computer. I don’t really care. But the form of intelligence that we might call general intelligence or adaptive intelligence, I do care about adaptive intelligence. I wonder how we can make machines where you can say, Here’s my problem, go solve it, in the way that you can tell an undergrad intern a few things about something and get them to go work on it and do some creditable work. We don’t have machines like that. We don’t have machines that have a kind of high-enough level of understanding of the world, or comprehension of the world, to be able to deal with novelty. Many of the examples you talk about are things where we have a massive amount of data that doesn’t change too much. So, you can get billions of trials of people saying the word “Alexa,” and then you can certainly use these algorithms to recognize the word “Alexa.”
Also: For a more dangerous age, a delicious skewering of current AI
On the other hand, Eric Topol, who’s one of my favorite people who works on AI and medicine, put a tweet out two days ago showing that there are serious problems still in getting AI to do anything really useful in medicine. And this is because biology is constantly changing.
To give you another case, a lot of these large language models think that Trump is still president because there’s lots of data saying President Trump, and they don’t do the basic temporal reasoning of understanding that once someone else is sworn in, that you’re not president anymore. They just don’t do that.
If you just accumulate statistical evidence and don’t understand the dynamics of things, you have a problem. Or, Walid Saba [AI and ML scientist] had this beautiful example. Who would you rather take advice from, he asked GPT-3, a young child or a brilliant table. And, it just knows the word brilliant, and so it says, I’d take the advice from the brilliant table. There’s no depth there, it’s not really understanding the world.
It’s a kind of brilliance but terror of marketing that the phrase deep learning implies conceptual depth, and that’s what it lacks. It actually only means a certain number of layers in the network, let’s say three or more, and nowadays it could be 150, but deep in deep learning just means number of layers, it does not mean conceptual depth. it does not mean that one of these systems knows what a person is, what a table is, what anything is.
ZDNET: Then it sort of seems that the forces against you are greater than the forces between you and LeCun. You’re both up against a regime in which things will be, as he put it, engineered. The world will achieve something that kind of works, but it really isn’t intelligent.
GM: It’s interesting: In a different world, LeCun and I would be allies. There’s a very large number of things that we agree on. I actually recently outlined them in a piece with the title, had the words in it, paradigm shift. I was actually responding to Slate Star Codex, Scott Alexander. I wrote a piece in my Substack, “Does AI really need a paradigm shift?” And there’s a section there in which I outline all the ways in which LeCun and I agree.
If you look at the larger texture of the field, we’re actually on most points in alignment. And I’ll review a few of them because I think they’re important. The No. 1 point on which LeCun and I are in alignment is that scaling alone is not enough. Now, we’re not alone in thinking that, but there’s a real schism in the field. I think a lot of the younger generation has been very impressed by the scaling demonstrations. [DeepMind researcher] Nando de Freitas wrote something on Twitter in which he said the game is over, AGI is just a matter of scaling. To which I wrote a reply called “Alt Intelligence,” which was the first piece in the Substack I’ve been keeping. People have been calling it scaling maximalism, lately, like scaling is all you need. That’s one of the biggest questions in the field right now. And LeCun and I are in absolute agreement that scaling maximalism, that that’s just not enough to get us to the kind of deeper adaptive intelligence that I think he and I both care about.
Similarly, he and I both think that reinforcement learning, which DeepMind has spent a lot of time on, but other people have as well, we also think that that’s inadequate. He likes to use the metaphor of “It’s just the cherry on top of the cake,” and I’m with him on that. I think you can’t do good reinforcement learning until you actually understand the world.
Also: AI Debate 2: Night of a thousand AI scholars
We both agree that large language models, although they’re really cool, are really problematic. Now, there I think I really pointed this out first, and he was really kind of vicious about it when I pointed it out. But we have converged on the same place. We both think that those systems, flashy as they are, are not getting us to general intelligence. And that’s related to the scaling point.
These are some of the most important issues. And in some sense, our collective view there is a minority view, and I believe that we’re both correct on those points. Time will tell. They’re all empirical questions. We have to do more work. We don’t know the scientific answers, but certainly LeCun and I share pretty deep intuitions around those points.
One other place where we really deeply agree, which is we deeply agree that you need to have models and common sense, it’s really two things. You need to have models of how the world works, and related to that, although we probably agree also that it’s nebulous, we both think that you need something like common sense and that that’s really critical.
I could imagine us sharing a panel at the World Science Festival, and then we would start to talk about, here are the seven things we agree with, and now here’s why I think world models need to be this way or that way, and it would be an interesting discussion if we could get back into that place where we once were.
ZDNET: And where you differ?
GM: I would make the case that there is a lot of symbolic knowledge that we might want to use. I would make the case that symbolic tools so far still offer a much better way of generalizing beyond the distribution, and that’s really important. We all nowadays know that distribution shift is a critical problem. I raised it in 2018, I think it’s still the essential problem, how you generalize beyond the data that you’ve seen. And I think symbolic models might have some advantage there. I would concede that we don’t know how to learn those models. And I think that LeCun’s best hope of making some advance there would be on the learning side of those models. I’m not sure he’s got the right architecture, but at least he has the right spirit in that sense.
Also: Devil’s in the details in historic AI debate
And then the other place where we substantively disagree, and this was the 2017 debate, which was about innateness. I think we need more innateness. And I think the great irony is that LeCun’s own greatest contribution to AI is the innate prior of convolution, which some people call translation invariance. And it says that, essentially, it’s a way of wiring in that an object is going to look the same if it appears in different locations. I think we need more priors like this. More innate stuff like this. And LeCun doesn’t really want it. He really doesn’t want there to be innate structure. He’s in the field called, not accidentally, machine learning. And people in machine learning want everything to be learned. Not all of them do, but many.
My view is if you look at biology that we are just a huge mix of innate structure and learned calibrational machinery. So, the structure of our heart, for example, is clearly innate. There’s some calibration. Your heart muscles can grow when you exercise, and so forth. But there’s lots and lots of innate structure. I find there to be a bias in the machine learning field against innateness that I think has really hurt the field and kept it back. So that’s a place where we would differ.
I do what I think people should do. I understand my opponent’s views. I think that I can characterize them and talk about points of agreement and disagreement and characterize what they are. Whereas what I think LeCun has been trying to do is to simply dismiss me off the stage. I don’t think that’s the right way to do science.
ZDNET: Exit question: What is it, do you think, that is important that we’re grappling with that is larger than both you and Yann LeCun?
GM: Well, I don’t think either of us has the answer, is the first thing I’ll say. And the reason I wish he would actually debate me ultimately is because I think that the field is stuck and that the only way we’re going to get unstuck is if some student or some young person sees things in a little bit different way than the rest of us have seen it. And having people like LeCun and myself who have strong points of view that they can articulate, can help people to see how to fix it. So, there is clearly great reason to want a learning-based system and not to want to hard-wire in. And there is clearly great reason to want the advantages of symbol manipulation. And there is no known way to sort of, as the saying goes, have our cake and eat it, too.
So, I like to think of there as being a space of possible models, right? Neural networks are all about exploring multi-dimensional spaces. There’s a space of possible architectures for AI. Most of what we’ve studied is in one little tiny corner of that space. That corner of the space is not quite working. LeCun and I actually agree about that. The question is, How do we get out of that corner and start looking at other places? And, we both have our guesses about it, but we certainly don’t know for sure. And there’s lots of room, I think, for many paradigm shifts left to come. In fact, in that piece of mine called “paradigm shift,” I quote LeCun as saying that. There is this part of the field that thinks we don’t need another paradigm shift, we just need more data. But LeCun and I both think that we do need paradigm shifts, which is to say we need to look outside the space of models that we’re looking at right now. The best way to help other people to do that is to articulate where we’re stuck.